The ROI of AI: Making the Business Case
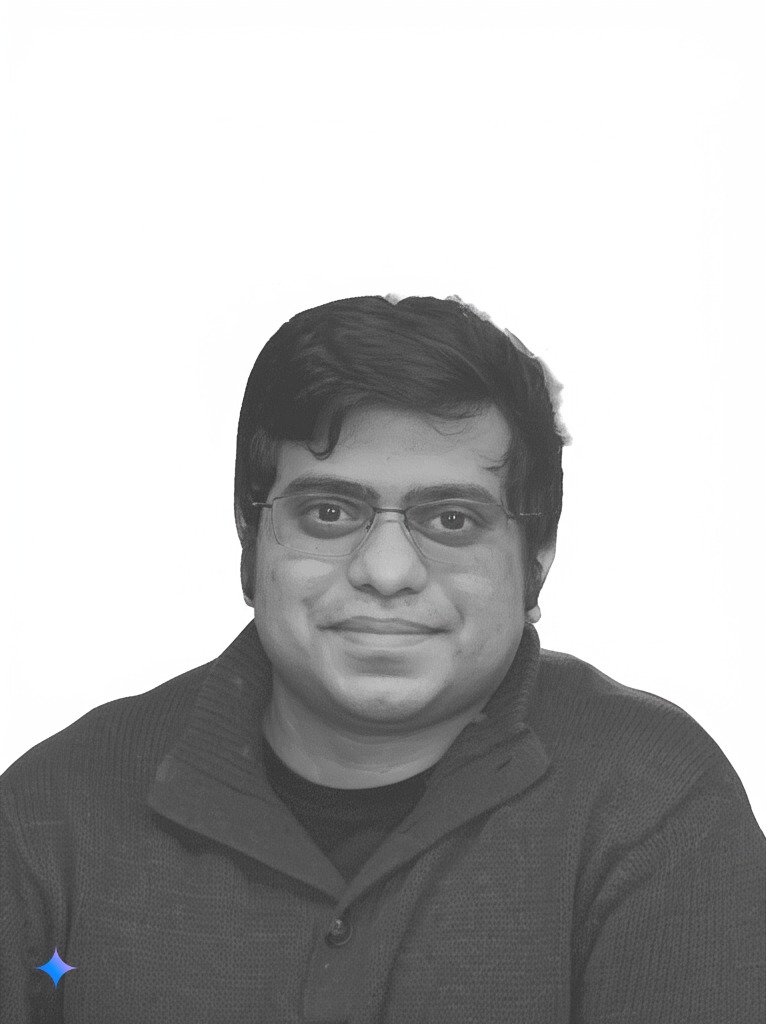
Saumil Srivastava
AI Consultant
Hey there,
Welcome to issue #40 of The AI Engineering Insider. This week, we're tackling a challenge that nearly every engineering leader faces: how to make a compelling business case for AI investments.
The ROI Challenge
Even as AI becomes mainstream, justifying the investment remains difficult. I consistently see three patterns that undermine AI business cases:
- Focusing solely on technical metrics (accuracy, F1 score) without connecting to business outcomes
- Underestimating the full cost of implementation, especially ongoing maintenance
- Over-promising on benefits, leading to unrealistic expectations and disappointment
I recently worked with a B2B SaaS company that had already invested $500K in an AI feature that customers barely used. The problem wasn't the technology—the model worked well—but they had failed to validate whether it solved a real customer problem worth paying for.
The 3-Layer ROI Framework
After helping dozens of companies make the business case for AI, I've developed a framework that addresses these common pitfalls:
1. Cost Modeling (Investment Layer)
Be comprehensive about costs:
- Development Costs: Engineering time, data collection/labeling, infrastructure
- Deployment Costs: Integration, testing, training
- Operational Costs: Monitoring, retraining, handling edge cases
- Opportunity Costs: What other initiatives are being delayed?
2. Value Identification (Impact Layer)
Map AI capabilities to specific business outcomes:
- Revenue Growth: Increased conversion, upsell, retention
- Cost Reduction: Automation, efficiency, error reduction
- Risk Mitigation: Fraud prevention, compliance, quality control
- Customer Experience: Satisfaction, engagement, NPS improvement
3. Proof Points (Validation Layer)
Evidence to support your projections:
- Internal Benchmarks: Results from pilots or similar initiatives
- Competitive Intelligence: Published results from competitors
- Industry Research: Case studies from similar implementations
- Customer Validation: Willingness to pay or usage data
This Week's Actionable Tip
Create a "Value Canvas" for your next AI initiative:
- List all stakeholders who would be affected by the AI system
- For each stakeholder, identify at least one quantifiable metric that matters to them
- Estimate the current baseline for each metric
- Project a realistic improvement range (conservative to optimistic)
- Translate improvements into financial impact where possible
A financial services client used this approach for their credit risk AI system. By identifying impacts across multiple departments (underwriting, collections, compliance), they uncovered 3x more potential value than their initial business case suggested.
What I'm Reading This Week
- "Prediction Machines: The Simple Economics of Artificial Intelligence" by Ajay Agrawal et al.
- "Measuring the Business Impact of AI" (McKinsey Global Institute Report)
That's all for this week! Next time, we'll dive into strategies for building reliable AI systems at scale.
Until then,
Saumil
P.S. Have you successfully made the case for an AI investment? I'd love to hear what worked for you.
Share this issue
Subscribe to The AI Engineering Insider
Get weekly insights on AI implementation, performance measurement, and technical case studies.
Join the Newsletter
Get weekly insights on AI implementation and technical case studies.